Related topics
The rapid rise of artificial intelligence has created an interesting paradox for modern enterprises.
While organizations race to adopt AI to drive innovation and efficiency, they simultaneously grapple with an important question: How can we ensure our AI systems operate responsibly and ethically in real-time?
This question isn't just theoretical—it's becoming increasingly critical as AI systems process massive amounts of sensitive data and make decisions that impact everything from healthcare outcomes to financial opportunities. As we’ll explore in this article, the answer lies in robust real-time AI monitoring, but the solution goes far deeper than simple performance metrics or periodic checks.

AI Running Wild
As AI systems become more integral to critical operations, they are making thousands of decisions that affect patient care, financial stability, and personal privacy. Traditional monitoring approaches—often relying on periodic reviews and manual checks—simply can't keep pace with the speed and complexity of modern AI operations.
This reality has pushed organizations to fundamentally rethink AI monitoring. Instead of treating it as an occasional checkpoint, leading enterprises are now embedding monitoring directly into their AI systems. This shift creates a continuous stream of insights about data flows, model behavior, and decision-making processes, allowing organizations to detect issues in real-time and respond proactively.
Breaking the Audit Loop
To address the challenges posed by AI's rapid decision-making capabilities, organizations are moving beyond basic monitoring techniques. Traditional audits and manual checks are no longer sufficient. Instead, there's a growing emphasis on sophisticated monitoring solutions that provide real-time visibility and control.
In runtime environments, advanced monitoring tools enable organizations to:
- Detect Anomalies: Identify unexpected behaviors or anomalies in AI model performance as they occur.
- Ensure Compliance: Validate that AI systems comply with regulatory standards and internal policies in real-time.
- Manage Third-Party Risks: Monitor interactions with third-party services and external data sources, where data flows can become complex and potentially problematic.
By adopting these advanced monitoring strategies, organizations not only enhance compliance but also gain the confidence to innovate more rapidly with AI technologies.
Unmasking AI Data
Understanding how AI systems transform raw data into derived insights is a significant challenge in AI monitoring. Traditional approaches often focus solely on raw inputs and outputs, overlooking the intricate data manipulations that occur within AI models. This oversight can create blind spots in data governance, leading to potential issues with privacy, compliance, and ethical use.
AI models frequently combine data from multiple sources to generate predictions or make decisions. While individual data points may appear harmless, their combination can reveal sensitive information or inadvertently introduce biases. For example, merging customer purchase histories with browsing behaviors can create detailed profiles that impact privacy.
Without advanced monitoring that tracks data lineage and transformations, organizations may unknowingly violate privacy regulations or fail to detect biases embedded in their AI systems. Real-time visibility into how data is processed and transformed is essential to identify and address these issues promptly.
Inside Modern Monitoring
Modern AI visibility tools provide comprehensive monitoring through a multi-layered approach. At the code level, organizations gain real-time insights into data processing logic and AI model behavior, enabling automated detection of potential privacy violations. Runtime monitoring tracks live data flows and transformations, immediately detecting unauthorized AI usage while validating privacy controls.
This monitoring extends to third-party integrations – a common blind spot in AI operations. Organizations can now track external data exchanges and prevent shadow AI proliferation while validating cross-border transfers and vendor compliance. This comprehensive visibility hasn't just improved compliance; it's enabled organizations to innovate more confidently with AI.
Privacy Without Paralysis
Privacy professionals face a distinct challenge in AI: ensuring compliance and data protection without hindering innovation. Two critical concerns dominate this space. First is the balancing act between data minimization and purpose limitation. Organizations must collect enough data for AI systems to function effectively while ensuring they don't exceed necessary scope. Modern monitoring tools turn this balancing act from guesswork into precision, enabling real-time verification of data collection and usage patterns.
Second is the changing nature of consent in AI systems. When data transforms and combines in unexpected ways, how do you ensure you're honoring original consent parameters? Real-time monitoring provides the granular visibility needed to track consent status and enforce requirements as data flows through AI systems.
Monitoring That Works
Successful implementation of real-time AI monitoring demands more than just deploying tools - it requires a structured, strategic approach. Organizations must carefully plan each phase while ensuring their monitoring framework aligns with both business objectives and compliance requirements.
1. Foundation Building: Foundation building starts with a clear understanding of your AI ecosystem. Organizations must document their AI systems, data flows, and processing activities. This baseline enables them to set meaningful monitoring metrics and implement tools that integrate naturally with existing infrastructure. More importantly, it helps establish clear protocols for when issues arise.
2. Continuous Oversight: Continuous oversight builds on this foundation through automated tools that track both AI behavior and data processing. Organizations can maintain clear visibility of how information transforms through AI systems while preventing shadow AI from taking root. Regular audit trails become a natural byproduct rather than a manual effort.
3. Risk Management: Risk management completes this framework by focusing on early detection. Rather than discovering privacy violations during periodic audits, organizations can identify and address issues as they emerge. This proactive approach helps maintain stakeholder trust while ensuring continuous compliance.
Building Resilient Monitoring
Effective real-time AI monitoring isn't just about implementing tools – it's about building a comprehensive approach that supports both innovation and governance. This means:
- Establishing clear visibility objectives that align with business goals and compliance requirements
- Implementing robust data lineage tracking that captures both direct and derived data relationships
- Developing automated compliance checks that can keep pace with AI operations
- Creating response protocols for when issues are detected
- Building feedback loops that support continuous improvement
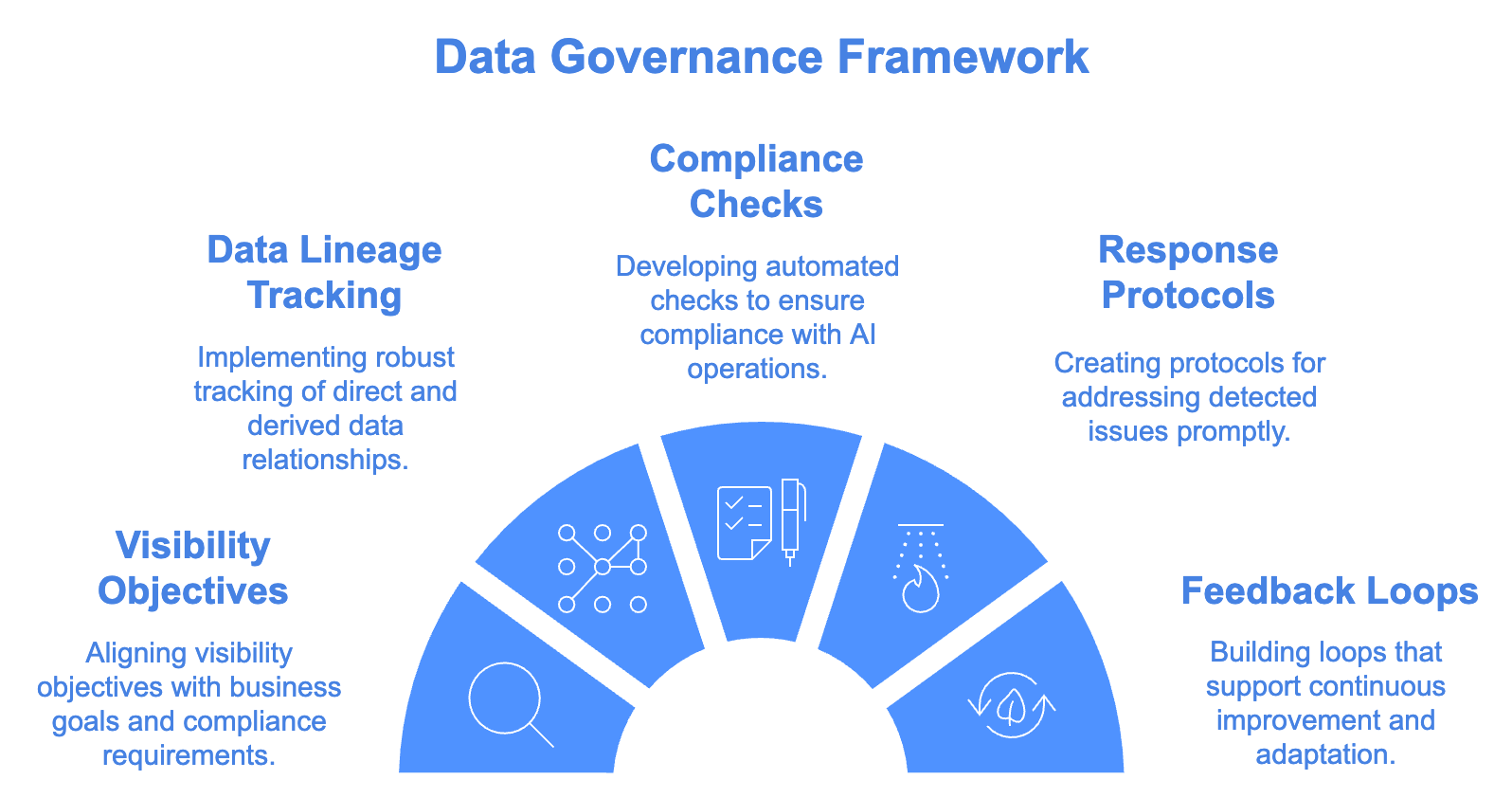
The impact of this approach extends beyond compliance. Organizations that implement comprehensive real-time monitoring often find they can innovate more confidently, knowing they have the visibility needed to ensure responsible AI operations.
The Next Wave
AI technology and privacy standards are evolving rapidly, pushing organizations to adapt their monitoring approaches. We're seeing a shift toward automated processes that can keep pace with AI system complexity. At the same time, privacy standards are becoming more stringent, particularly around shadow AI prevention and detection.
Organizations that implement comprehensive monitoring solutions today aren't just checking compliance boxes – they're building the foundation for responsible AI innovation. The focus must remain on developing approaches that scale with new requirements while delivering insights that drive action.
Time for Action
Real-time AI monitoring has become a strategic necessity rather than a technical nice-to-have. Organizations that understand this shift are implementing robust visibility tools and continuous tracking mechanisms that do more than prevent shadow AI – they enable confident innovation while maintaining compliance and trust.
The future belongs to organizations that can effectively monitor their AI systems in real-time, ensuring responsible innovation while maintaining effective governance. Those who invest in comprehensive monitoring solutions today are building the foundation for trusted AI operations tomorrow.